The Rise of Edge AI: Bringing AI to the Edge Devices
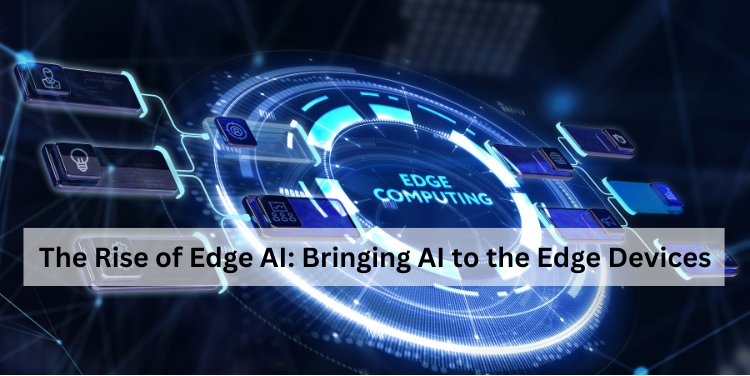
Artificial Intelligence (AI) has revolutionized numerous industries by providing intelligent solutions to complex problems. Traditionally, AI processing has been centralized, relying on powerful cloud-based servers to perform heavy computations. However, a new paradigm is emerging: Edge AI. This innovative approach brings AI capabilities directly to edge devices such as smartphones, IoT devices, and industrial equipment, enabling faster, more efficient, and secure processing. In this blog post, we'll explore the rise of Edge AI and its transformative impact on technology and industry.
What is Edge AI?
Edge AI refers to the deployment of AI algorithms and models on edge devices, which are located closer to the data source rather than relying on centralized cloud servers. These devices can process data locally, making real-time decisions and actions possible without needing to send data to the cloud. This shift towards edge computing is driven by several factors, including the need for low-latency responses, enhanced privacy and security, and reduced bandwidth usage.
The Advantages of Edge AI
-
Reduced Latency: One of the primary benefits of Edge AI is reduced latency. By processing data locally on the device, Edge AI can deliver real-time responses that are critical for applications such as autonomous vehicles, industrial automation, and augmented reality. This low-latency capability is crucial for scenarios where even a slight delay can have significant consequences.
-
Enhanced Privacy and Security: With Edge AI, sensitive data can be processed locally, reducing the need to transmit it over the internet to cloud servers. This localized processing enhances privacy and security by minimizing the risk of data breaches and unauthorized access. Edge AI is particularly beneficial for applications in healthcare, finance, and other sectors that handle sensitive information.
-
Bandwidth Efficiency: Edge AI reduces the reliance on constant internet connectivity and minimizes the amount of data that needs to be sent to the cloud. This efficiency is especially valuable in environments with limited bandwidth or intermittent connectivity. By processing data locally, Edge AI can significantly reduce the strain on network resources.
-
Cost Savings: By offloading processing tasks from cloud servers to edge devices, organizations can achieve cost savings in terms of cloud infrastructure and data transfer fees. Additionally, the reduced need for constant internet connectivity can lower operational costs.
Applications of Edge AI
-
Autonomous Vehicles: Autonomous vehicles require real-time processing of sensor data for tasks such as object detection, navigation, and collision avoidance. Edge AI enables these vehicles to make split-second decisions, enhancing safety and performance.
-
Smart Cities: Edge AI plays a crucial role in smart city applications, including traffic management, surveillance, and environmental monitoring. Localized processing allows for real-time analysis and response to dynamic urban environments.
-
Healthcare: In healthcare, Edge AI can be used for remote patient monitoring, diagnostic imaging, and personalized treatment plans. By processing data locally, healthcare providers can deliver timely interventions and improve patient outcomes.
-
Industrial Automation: Edge AI enhances industrial automation by enabling real-time monitoring and predictive maintenance of machinery and equipment. This capability reduces downtime, increases efficiency, and extends the lifespan of assets.
-
Consumer Electronics: Edge AI is becoming increasingly prevalent in consumer electronics, such as smartphones, wearables, and smart home devices. Features like voice recognition, image processing, and contextual awareness are made possible through on-device AI processing.
Challenges and Future Outlook
While Edge AI offers numerous advantages, it also presents challenges. Edge devices typically have limited computational power and memory compared to cloud servers, necessitating efficient algorithms and optimized models. Additionally, managing and updating AI models across a diverse array of edge devices can be complex.
However, advancements in hardware, such as specialized AI chips and improved energy efficiency, are addressing these challenges. The future of Edge AI looks promising, with continued innovation driving its adoption across various industries.
Edge AI represents a significant shift in how AI is deployed and utilized. By bringing AI capabilities closer to the data source, Edge AI offers reduced latency, enhanced privacy, bandwidth efficiency, and cost savings. Its applications span numerous sectors, from autonomous vehicles to healthcare, smart cities, industrial automation, and consumer electronics. As technology continues to evolve, Edge AI is poised to play a pivotal role in shaping the future of intelligent computing. Embracing this paradigm will unlock new possibilities and drive further advancements in AI and beyond.
What's Your Reaction?
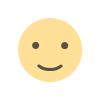
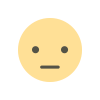
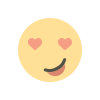
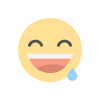
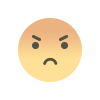
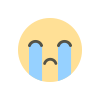
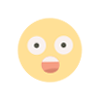